- New
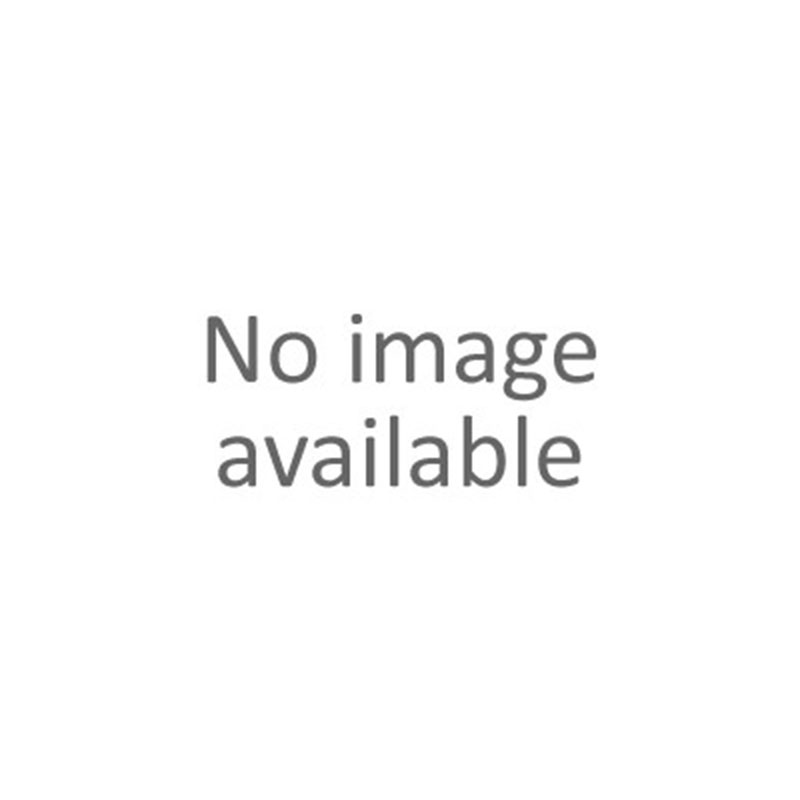
References Bai, T., Tahmasebi, P., Graph neural network for groundwater level forecasting. J. Hydrology, 616 (2023) 128792. https://doi.org/10.1016/j.jhydrol.2022.128792 Fang, X., Wu, J., Jiang, P., Liu, K., Wang, X., Zhang, S., Lai, Y., A rapid assessment method for flood risk mapping integrating aerial point clouds and deep learning. Wat. Resour. Manage., 38 (2024) 1753–1772. https://doi.org/10.1007/s11269-024-03764-5 Gil-Gamboa, A., Paneque, P., Trull, O., Troncoso, A., Medium-term water consumption forecasting based on deep neural networks. Expert Syst. Applic., 247 (2024) 123234. https://doi.org/10.1016/j.eswa.2024.123234 Ji, H.K., Mirzaei, M., Lai, S.H., Dehghani, A., Dehghani, A., Implementing generative adversarial network (GAN) as a data-driven multi-site stochastic weather generator for flood frequency estimation. Environ. Model. Software, 172 (2024) 105896. https://doi.org/10.1016/j.envsoft.2023.105896 Kao, I.F., Zhou, Y., Chang, L.C., Chang, F.J., Exploring a long short-term memory based encoder-decoder framework for multi-step-ahead flood forecasting. J. Hydrology, 583 (2020) 124631. https://doi.org/10.1016/j.jhydrol.2020.124631 Li, B., Li, R., Sun, T., Gong, A., Tian, F., Khan, M.Y.A., Ni, G. Improving LSTM hydrological modeling with spatiotemporal deep learning and multi-task learning: A case study of three mountainous areas on the Tibetan Plateau. J. Hydrology, 620 A (2023) 129401. https://doi.org/10.1016/j.jhydrol.2023.129401 Liu, P., Wang, J., Sangaiah, A.K., Xie, Y., Yin, X. Analysis and prediction of water quality using LSTM deep neural networks in IoT environment. Sustainability, 11(7) (2019) 2058. https://doi.org/10.3390/su11072058 Rajabi, M. M., Javaran, M. R. H., Bah, A. O., Frey, G., Le Ber, F., Lehmann, F., Fahs, M., Analyzing the efficiency and robustness of deep convolutional neural networks for modeling natural convection in heterogeneous porous media. Int. J. Heat Mass Transfer, 183 (2022) 122131. https://doi.org/10.1016/j.ijheatmasstransfer.2021.122131 Rajabi, M.M., Komeilian, P., Wan, X., Farmani, R., Leak detection and localization in water distribution networks using conditional deep convolutional generative adversarial networks. Water Res., 238 (2023) 120012. https://doi.org/10.1016/j.watres.2023.120012 Ren, X., Li, X., Ren, K., Song, J., Xu, Z., Deng, K., Wang, X., Deep learning-based weather prediction: a survey. Big Data Res., 23 (2021) 100178. https://doi.org/10.1016/j.bdr.2020.100178 Samek, W., Montavon, G., Lapuschkin, S., Anders, C.J., Müller, K.R., Explaining deep neural networks and beyond: A review of methods and applications. Proc. IEEE, 109(3) (2021) 247–278. https://doi.org/10.1109/JPROC.2021.3060483 Shen, C., A transdisciplinary review of deep learning research and its relevance for water resources scientists. Water Resour. Res., 54(11) (2018) 8558–8593. https://doi.org/10.1029/2018WR022643 Stoian, A., Poulain, V., Inglada, J., Poughon, V., Derksen, D., Land cover maps production with high resolution satellite image time series and convolutional neural networks: Adaptations and limits for operational systems. Remote Sensing, 11(17) (2019) 1986. https://doi.org/10.3390/rs11171986 Wang, J., Pang, X., Yin, F., Yao, J., A deep neural network method for solving partial differential equations with complex boundary in groundwater seepage. J. Petrol. Sci. Eng., 209 (2022) 109880. https://doi.org/10.1016/j.petrol.2021.109880
References Bai, T., Tahmasebi, P., Graph neural network for groundwater level forecasting. J. Hydrology, 616 (2023) 128792. https://doi.org/10.1016/j.jhydrol.2022.128792 Fang, X., Wu, J., Jiang, P., Liu, K., Wang, X., Zhang, S., Lai, Y., A rapid assessment method for flood risk mapping integrating aerial point clouds and deep learning. Wat. Resour. Manage., 38 (2024) 1753–1772. https://doi.org/10.1007/s11269-024-03764-5 Gil-Gamboa, A., Paneque, P., Trull, O., Troncoso, A., Medium-term water consumption forecasting based on deep neural networks. Expert Syst. Applic., 247 (2024) 123234. https://doi.org/10.1016/j.eswa.2024.123234 Ji, H.K., Mirzaei, M., Lai, S.H., Dehghani, A., Dehghani, A., Implementing generative adversarial network (GAN) as a data-driven multi-site stochastic weather generator for flood frequency estimation. Environ. Model. Software, 172 (2024) 105896. https://doi.org/10.1016/j.envsoft.2023.105896 Kao, I.F., Zhou, Y., Chang, L.C., Chang, F.J., Exploring a long short-term memory based encoder-decoder framework for multi-step-ahead flood forecasting. J. Hydrology, 583 (2020) 124631. https://doi.org/10.1016/j.jhydrol.2020.124631 Li, B., Li, R., Sun, T., Gong, A., Tian, F., Khan, M.Y.A., Ni, G. Improving LSTM hydrological modeling with spatiotemporal deep learning and multi-task learning: A case study of three mountainous areas on the Tibetan Plateau. J. Hydrology, 620 A (2023) 129401. https://doi.org/10.1016/j.jhydrol.2023.129401 Liu, P., Wang, J., Sangaiah, A.K., Xie, Y., Yin, X. Analysis and prediction of water quality using LSTM deep neural networks in IoT environment. Sustainability, 11(7) (2019) 2058. https://doi.org/10.3390/su11072058 Rajabi, M. M., Javaran, M. R. H., Bah, A. O., Frey, G., Le Ber, F., Lehmann, F., Fahs, M., Analyzing the efficiency and robustness of deep convolutional neural networks for modeling natural convection in heterogeneous porous media. Int. J. Heat Mass Transfer, 183 (2022) 122131. https://doi.org/10.1016/j.ijheatmasstransfer.2021.122131 Rajabi, M.M., Komeilian, P., Wan, X., Farmani, R., Leak detection and localization in water distribution networks using conditional deep convolutional generative adversarial networks. Water Res., 238 (2023) 120012. https://doi.org/10.1016/j.watres.2023.120012 Ren, X., Li, X., Ren, K., Song, J., Xu, Z., Deng, K., Wang, X., Deep learning-based weather prediction: a survey. Big Data Res., 23 (2021) 100178. https://doi.org/10.1016/j.bdr.2020.100178 Samek, W., Montavon, G., Lapuschkin, S., Anders, C.J., Müller, K.R., Explaining deep neural networks and beyond: A review of methods and applications. Proc. IEEE, 109(3) (2021) 247–278. https://doi.org/10.1109/JPROC.2021.3060483 Shen, C., A transdisciplinary review of deep learning research and its relevance for water resources scientists. Water Resour. Res., 54(11) (2018) 8558–8593. https://doi.org/10.1029/2018WR022643 Stoian, A., Poulain, V., Inglada, J., Poughon, V., Derksen, D., Land cover maps production with high resolution satellite image time series and convolutional neural networks: Adaptations and limits for operational systems. Remote Sensing, 11(17) (2019) 1986. https://doi.org/10.3390/rs11171986 Wang, J., Pang, X., Yin, F., Yao, J., A deep neural network method for solving partial differential equations with complex boundary in groundwater seepage. J. Petrol. Sci. Eng., 209 (2022) 109880. https://doi.org/10.1016/j.petrol.2021.109880