- New
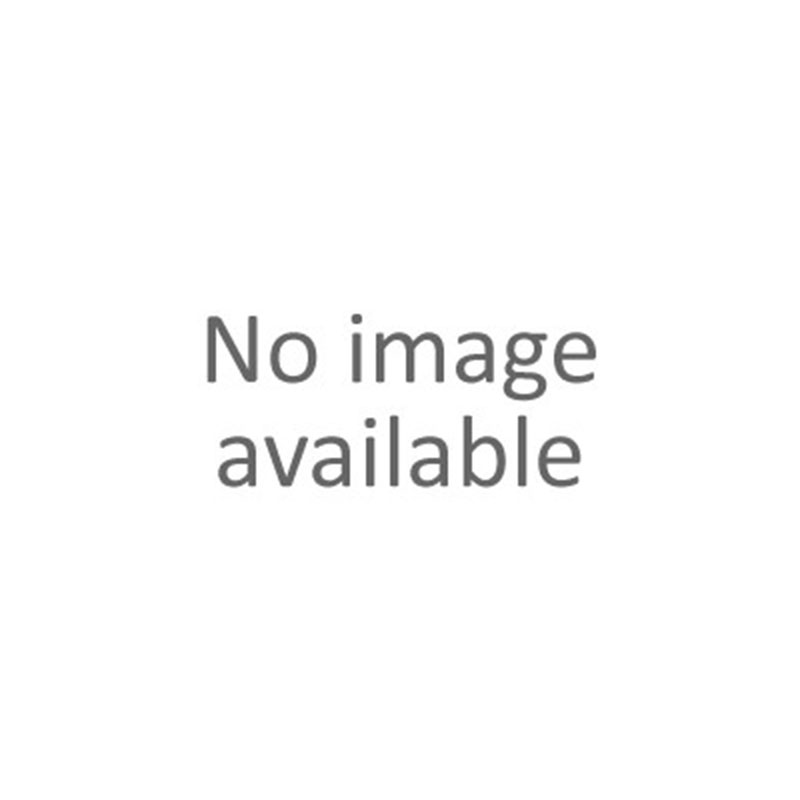
Traditionally, environmental and water resources simulations (EWRS) have relied on physics-based analytical and numerical models. These models employ parameters that characterize the environmental systems, system state variables, and external forces as input into mathematical equations to predict future conditions of environmental systems and water resources. The effectiveness of these models is frequently limited due to the considerable computational resources and lengthy simulation times required for large-scale or repetitive simulations, and the partial comprehension or flawed mathematical representation of the physical processes that result in a mismatch between the predicted outcomes of the models and real-world observations at the field scale (Rajabi et al., 2023). To address these challenges, there has been a shift towards employing data-driven models that incorporate machine learning (ML) techniques. Compared to traditional physics-based models, ML models are typically faster, simpler to develop, and require less detail information. Historically, a range of ML tools have been applied to develop data-driven models for EWRS, including random forests, support vector machines, polynomial chaos expansion, and tree-based regression models. Nevertheless, conventional ML methods often face difficulties when encountering infrequent, black swan cases within the dataset, struggle to adapt to new scenarios not included in their training data, may not effectively manage large volumes of data, and fall short in identifying the deep relationships and complex patterns among the parameters that affect outcomes. Deep neural networks (DNNs), a newer segment of ML, provide more flexibility and have shown to offer higher accuracy in predictions, particularly with extensive datasets (Samek et al., 2021). Their advanced learning capacities make DNNs a highly researched tool for EWRS, demonstrating significant promise over classical ML techniques.
Traditionally, environmental and water resources simulations (EWRS) have relied on physics-based analytical and numerical models. These models employ parameters that characterize the environmental systems, system state variables, and external forces as input into mathematical equations to predict future conditions of environmental systems and water resources. The effectiveness of these models is frequently limited due to the considerable computational resources and lengthy simulation times required for large-scale or repetitive simulations, and the partial comprehension or flawed mathematical representation of the physical processes that result in a mismatch between the predicted outcomes of the models and real-world observations at the field scale (Rajabi et al., 2023). To address these challenges, there has been a shift towards employing data-driven models that incorporate machine learning (ML) techniques. Compared to traditional physics-based models, ML models are typically faster, simpler to develop, and require less detail information. Historically, a range of ML tools have been applied to develop data-driven models for EWRS, including random forests, support vector machines, polynomial chaos expansion, and tree-based regression models. Nevertheless, conventional ML methods often face difficulties when encountering infrequent, black swan cases within the dataset, struggle to adapt to new scenarios not included in their training data, may not effectively manage large volumes of data, and fall short in identifying the deep relationships and complex patterns among the parameters that affect outcomes. Deep neural networks (DNNs), a newer segment of ML, provide more flexibility and have shown to offer higher accuracy in predictions, particularly with extensive datasets (Samek et al., 2021). Their advanced learning capacities make DNNs a highly researched tool for EWRS, demonstrating significant promise over classical ML techniques.